New Release
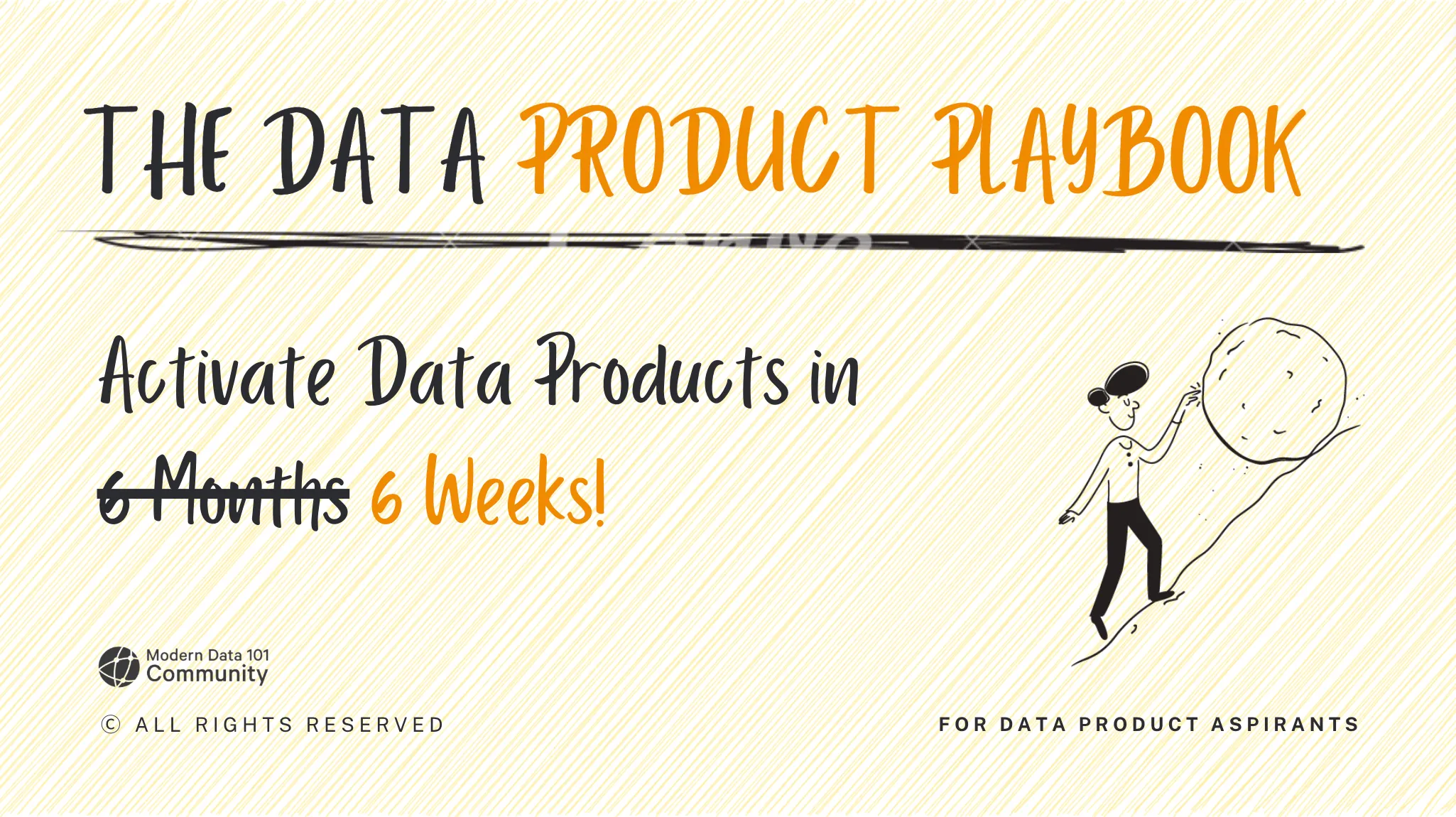
Learn More
Your submission has been received!
Thank you for submitting!
Thank you for submitting!
Download your PDF
Oops! Something went wrong while submitting the form.
Table of Contents
Get weekly insights on modern data delivered to your inbox, straight from our hand-picked curations!
the following is a revised edition.
The Stepping Stone: The Traditional Data Stack and why it became obsolete
The Obvious Next Step: The Modern Data Stack & the MAD ecosystem
The Solution: The Data-First Stack
Defining Factors of a Data-First Stack
Outcome of a Data-First Stack
Summary
If you have been even remotely related to the data space, I can bet you’ve already experienced the nuances of the chaotic data ecosystem. There is no doubt that the data world has gone through its own share of evolution and has, in fact, come a long way.
Today, it is nearly unimaginable to manually write, read, and manage data, even for small companies, due to the sheer magnitude of data generation. Every click, every view, and every event has significant and potentially beneficial business results if the patterns are identified successfully.
Imagine these events multiplied several-fold, at the scale of, say, millions or even billions of events. How do you process this volume and ensure the data is not just taking up expensive space but showing value for its existence?
The data stack has been under a constant state of movement to accommodate the pace at which data has grown. Actually, it is not the data that has grown, data is ever present in the ether. The growth has been in our technological capabilities that have, over time, evolved to capture various streams of data. For instance, IoT devices today even detect breath and movements to moderate air conditioning capabilities.
However, while the sensory abilities to capture and record data have grown significantly, the abilities to process, manage and understand that data have not progressed at the same rate. So yes, we have the eyes, ears, skin, tongue, and nose, but we still lack a well-formed brain that can understand and operationalize the inputs from these channels.
Data has always been present, and there will be no dearth of data generation even in the future. We, as an industry, understood that this ever-present data could and should be leveraged to optimize returns on resources and spend.
🔑 A key point to note here is that the primary objective of leveraging data was and will be to enrich the business experience and returns.
To enable data operationalization, the first concrete stepping stone was the Traditional Data Stack. Well, at that point, it was not “traditional”; it was THE data stack. That somewhat changes the perspective, isn’t it? A decade or two down the line, the prevalent stacks today could easily fall back into the traditional or legacy bucket.
The traditional data stack (TDS), simply put, is another name for on-prem data systems. Organizations managed their own infrastructure and hardware that was not just manual-intensive but also a burden in terms of fragility (change resistance), high cost of maintenance, lack of scalability (provision new infra or h/w every time the stack needs to scale), rigidity due to bottom-up maintenance, development from scratch, and extensively complicated root cause analysis or the lack of it.
Since the components in a TDS, be it warehouses or big data clusters, are tightly coupled with each other, it is extremely difficult to decouple logical requirements from raw physical data, thereby slowing down business, recovery, and RCA capabilities.
Being manual-intensive and tightly coupled is also the reason behind the high fragility of the TDS and the data pipelines it supports. Transformation jobs are not just slow due to the archaic technology but also due to the unstable pipelines that break on encountering dynamic data or events, and as we all know, data is ever-changing.
And, of course, coming to the core problem. The whole objective of a data stack is to facilitate business. The cost incurred to maintain and scale the TDS is a significant hit on the data team’s ROI. Even if we assume the value produced by the stack is useful and reaches the business teams at the right time, the expense of ensuring that transition eats up over half of the value.
As a result, TDS has always been far from being an A-player when it comes to performance in terms of quality, volume, and especially time sensitivity & ROI, which are key for businesses. But something was better than nothing and it got us to see finer problems that were a level up from the problems of data stored in heaps of physical files and folders, sitting dormant somewhere deep in the basement.
It is what it is. We cannot deny the impressive evolution the Modern Data Stack (MDS) has brought around from the state of TDS. The biggest achievement has perhaps been the revolutionary shift to the cloud, which has made data not just more accessible but also recoverable.
🧩 The Modern Data Stack is a collection of multiple point solutions that are stitched together by users to enable an active flow from physical data to business insights. We have all seen the hype around MDS and how it engrossed the data community in potential and possibilities.
But what really happened was that the MDS came up as a disconnected basket of solutions that targeted patches of the TDS problem with overwhelmed pipelines and dumped all data to a central lake that eventually created unmanageable data swamps across industries.
Big picture, data swamps are no better than physical files in the basement. Data swamps are clogged with rich, useful, yet dormant data that businesses are unable to operationalize due to isolated and untrustworthy semantics around that data.
Semantical untrustworthiness stems from the chaotic clutter of MDS, where there are so many tools, integrations, and unstable pipelines that the true and clean semantics gets lost in the web. Another level of semantics is required to understand the low-level semantics, which just complicates the problem further.
Chaos has ensued for non-expert end users as data ecosystems progressively develop into complex and siloed systems with a continuous stream of point solutions added to the insane mix every other day. No surprise it has been called the MAD (ML, AI, & Data) landscape. This infographic by Matt Turck instantly points out the issue with MDS; no words needed (a picture is worth a thousand words!).
The MADness manifests in terms of a dilemma of choices, integration overheads, maintenance overheads, expertise overheads, and resource overheads. The chaotic ecosystem of countless point solutions ends up creating data silos instead of solving them.
To understand how the MDS has become a burden for organizations, feel free to jump to “The Weight of the Modern Data Stack.”
As opposed to MDS
The emergence of the Modern Data Stack (MDS) led us to overcome the resistance of on-prem and manual-intensive challenges. However, the MDS also turned out to be a double-edged sword that brought new problems into the limelight, even ones that we didn’t anticipate while the MAD tree grew one leaf at a time.
The Data-First Stack (DFS) is a milestone innovation that is inspired by the data-first movement undertaken by a couple of data-first organizations such as Uber, Google, and Airbnb over the last decade. But what does data-first mean?
Data-first, as the name suggests, is putting data and data-powered decisions first while de-prioritizing everything else either through abstractions or intelligent design architectures. It would be easier to understand this if we look at it from the opposite direction - “data-last”.
Current practices, including MDS, is an implementation of “data-last” where massive efforts, resources, and time are spent on managing, processing, and maintaining data infrastructures. Data and data applications are literally lost in this translation and have become the last focus points for data-centric teams, creating extremely challenging business minefields for data producers and data consumers.
Time to ROI (TTROI)
During the last decade, it took organizations several years to build a data-first stack due to the state of technology, limited innovations, and especially because we had very less insight or understanding of the dataverse.
Today, businesses that have a good grasp of data make the difference between winning and losing the competitive edge. Many data-first organizations understood this long back and dedicated major projects to becoming data-first. However, replicating them is not the solution since their data stacks were catered to their specific internal architectures.
🔑 A data-first stack is only truly data-first if it is built in accordance with your internal infrastructure.
Contrary to the widespread mindset that it takes years to build a data-first stack, with new storage-and-compute tools and innovative technologies that have popped up in the last couple of years, this is no longer true. It is not impossible to build a data-first stack and reap value from it within weeks instead of months and years.
Non-Disruptive
The easy transition to DFS is feasible since a data-first stack is not disruptive as it does not aim to rip and replace existing tools or massive data investments that came prior to it. It facilitates existing design architectures by providing a unified control plane on top of complex subsystems. Over time, these subsystems could be replaced with DFS native building blocks based on the user’s ease of transition or preference
Quoting Martin Fowler on this one: “This situation is counter to our usual experience. We are used to something that is "high quality" as something that costs more. But when it comes to the architecture, and other aspects of internal quality, this relationship is reversed. High internal quality leads to faster delivery of new features because there is less cruft to get in the way.”
A unified approach mercilessly trims the moving parts that plug into the data ecosystem. More tools bring in more cruft (debt) and make the problem more complex. A unified approach composes the functionality to enable a single management plane.
The key to creating a truly unified architecture is to declutter and instead have a minimalistic set of building blocks. These blocks could be put together in any necessary sequence to build larger and finer solutions that have loosely coupled and tightly integrated components.
A unified architecture powers very specific data applications with just a few degrees of rearrangement. The ultimate goal is to have curated self-service layers that abstract users from the complexities of complex, siloed, and isolated subsystems so they can focus on the problem at hand - the data.
A truly Data-First Stack has a laser focus on data and data applications instead of diversifying efforts across underlying operations such as ingestion, integrations, orchestration, low-level storage nuances, etc. Moreover, data engineering teams spend more time fixing pipelines than creating new ones to power business opportunities. A declaratively managed system eliminates the scope of fragility dramatically and surfaces RCA lenses on demand.
Declarative management of data quality, governance, security, and semantics was considered an impossibility, but thanks to the latest revolutionizing idea of data contracts, declarative capabilities in the data landscape could be easily materialized. The best part is that contracts do not disrupt any existing infrastructures.
🔶 Quick refresher on contracts
Data contracts are expectations on data. These expectations can be in the form of business meaning, data quality, or data security. It is an agreement between data producers and data consumers that documents and declaratively ensures the fulfillment of data expectations.
Watch this space for a deeper dive into contracts soon.
A data developer platform with a unified architecture ideology laced with contractual handshakes is the holy grail for a declarative data ecosystem and, consequently, the true enabler of a data-first stack.
A Data-First Stack’s primary end-user is unquestionably the Data Developer. The data developer’s experience while working with data and building data apps is a game changer for resource optimization and business timelines. A data-first stack promotes developer experience and abstracts low-level resource management tasks while not compromising their flexibility to allow data developers the complete freedom to declaratively manage less strategic operations.
Contracts are playing a key role in curating the optimal developer experience as well. As David Jayatillake put it, “we need to end the disaster of Data Engineering without Data Contracts as an industry.” Where does the data contract optimally fit in? And why should Data Engineers leave thousands of pending requests to consider the impact of contracts on their day-to-day experience?
With the simple philosophy of contractual handshakes distributed across the data landscape, contracts almost perfectly align with the Value Pyramid (published by Harvard Business Review, 2016) that:
Full disclosure on a Data-First stack is that the initial climb is slow, but once the initial weeks are overcome, the value is instantly realized since a truly data-first stack goes exactly by its name: It puts data and metrics first and ties processes directly to business outcomes. If you think about it, Data-First is synonymous with outcome-first.
The end objective of a data-first stack is to create Data Products. Even though this should be the ideal objective of any data stack, data team, or data initiative, the end goal of creating valuable data that actually uplifts business objectives has somehow been lost among the complexities of prevalent data stacks.
The data-first stack clears out the distractions to bring back focus on data and data applications. When data is channeled through the components of a data-first stack, the typical output is a data product which, simply put, is a unit of data that adds value to the user consistently and reliably. A data product has a few distinct qualities or attributes that differentiate it from general data.
A data product, if imbibing the above qualities, could easily be a simple spreadsheet, files in an S3 bucket, a table, a database, features stored in ML feature stores… you get the idea.