New Release
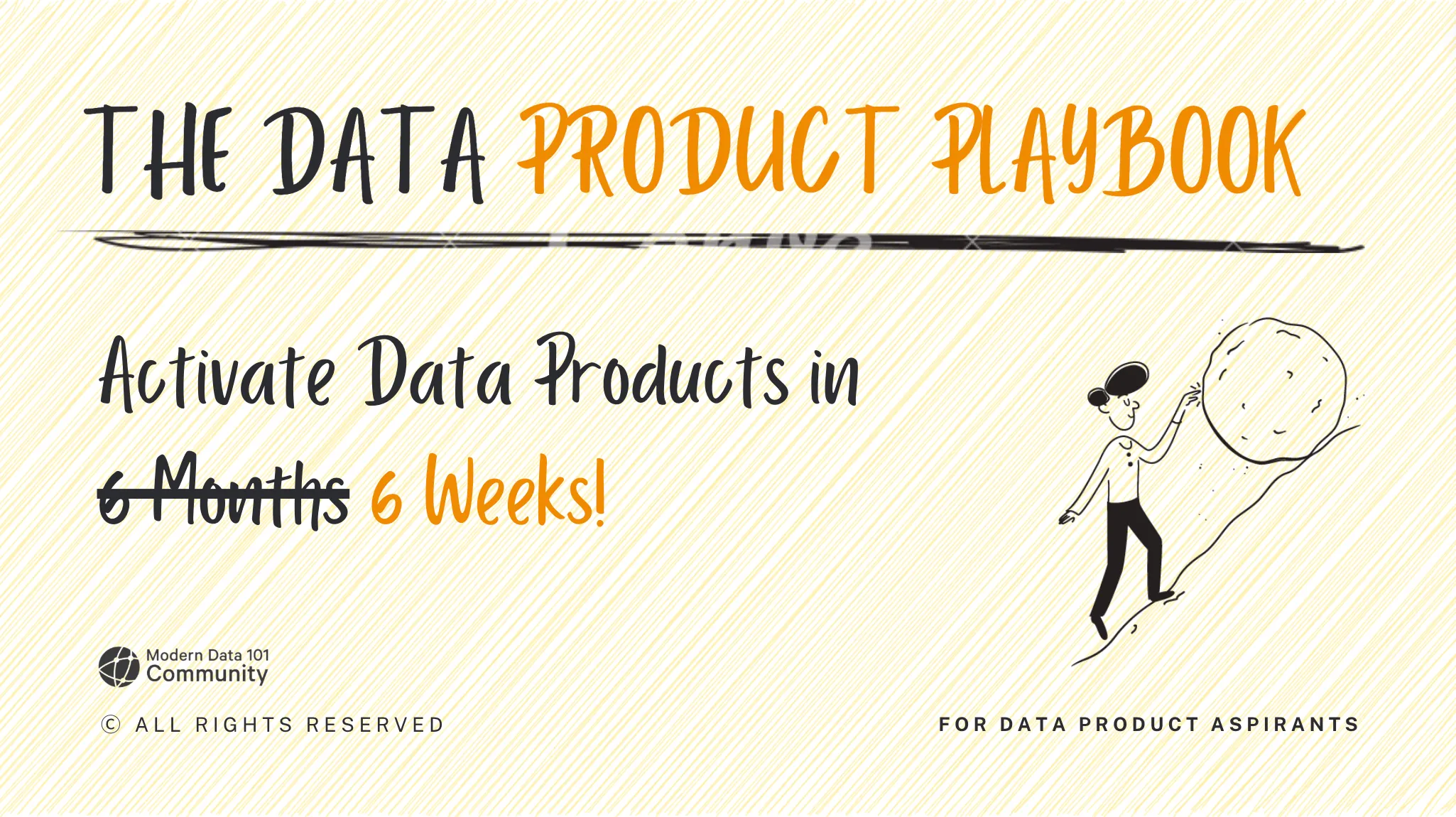
Learn More
Your submission has been received!
Thank you for submitting!
Thank you for submitting!
Download your PDF
Oops! Something went wrong while submitting the form.
Table of Contents
Get weekly insights on modern data delivered to your inbox, straight from our hand-picked curations!
the following is a revised edition.
Artificial Intelligence (AI) has rapidly become a transformative force across industries, promising to revolutionize everything from customer experiences to operational efficiency ⚡️
However, the success of AI initiatives is not guaranteed– it requires more than just cutting-edge algorithms and large datasets (though those are important, too!). Poorly executed AI deployments can lead to unreliable outcomes, wasted resources, huge compute bills, and even reputational damage.
To harness the full potential of AI while minimizing risks, organizations must focus on foundational elements like data quality, governance, and infrastructure. This article delves into the key considerations for avoiding these pitfalls.
AI is cool. But….a failed AI project wastes time, resources, money, and even puts a company’s reputation at risk.
Remember last year when two of the nation’s largest health insurers, Cigna and United Healthcare (UHC), allegedly crossed the line by integrating AI predictive tools into their systems to automate claim denials for medical necessity, improperly denying patients health care coverage for medical services and overriding the medical determinations of their doctors.
More alarmingly, ethical missteps in AI deployment—such as biased algorithms or privacy violations—can lead to severe legal repercussions and loss of customer trust. Not to mention the new pending regulations around AI, like the AI Act in Europe. Even X’s (formerly Twitter’s) AI plans have been hit with 9 more GDPR complaints!
These potential risks underscore the importance of a strategic approach to AI implementation.
But WHY does AI fail? Many people get caught up in the hype about what the potential outcome of using AI could be (think about how quickly ChatGPT became a “thing”), but many forget to take a step back and consider how to make sure their [typically large] investments in AI are effective and safe.
Several factors can lead to the failure of AI projects:
Effectively deploying AI requires careful planning, continuous stakeholder engagement, and a robust testing and data management framework. By learning from successful deployments and avoiding common pitfalls, organizations can harness the full potential of AI technologies while minimizing risks.
As AI continues to evolve, the businesses that thrive will be those that integrate AI thoughtfully and strategically into their operations.
AI models are only as good as the data they are trained on. Your AI outputs will be flawed if your data is inaccurate, incomplete, or poorly structured. AI boils down to an equation that computes on values provided (data).
The Challenges of Building a Strong Data Foundation ⬇️
A strong data foundation is critical, encompassing data quality, availability, and integrity. Organizations should prioritize establishing reliable data pipelines and governance practices that ensure data is clean, compliant, enriched, and up-to-date before it even reaches AI training pipelines. It also requires ongoing monitoring of data quality to detect and address any issues that arise over time.
Re-emphasizing, AI models are only as good as the data they are trained on. This truth underpins the success of any AI deployment. Poor data quality—characterized by inaccuracies, biases, inconsistencies, and incompleteness—can lead to unreliable AI outputs. If the data fed into an AI model is flawed, the predictions, decisions, or recommendations made by that model will be equally flawed.
It’s important to note that quality, availability, and other governance requirements can vary across teams/users and AI agents, even for the same data. A Product approach for Data helps engineering teams deliver data with these requirements in mind (right-to-left data development)
Ergo, a data “Product” furnishes a range of experience ports, each with its own SLOs (quality & governance objectives) - essentially allowing producers to meet where the user is: native environment, comfortable consumption patterns or formats, and necessary quality conditions.
🔖 Related Reads
If you wish to deep-dive, here are more detailed resources on building strong data foundations:
1. How Data Becomes Product
2. How to Build Data Products - Design: Part 1/4
3. Essence of Having Your Own Data Developer Platform
.
👁️🗨️ A Quick Visual Example
Consider a use case where you want to build LLMs for your business teams or add GPT offerings to customer interfaces. Without the correct context and data served by the underlying layers, the AI experience downstream would be broken, cause frustrations, or even add to high-cost damages (reputational or legal).
This is where a strong data foundation takes effect by solving the data irregularities below, adding more context to AI to deliver more reliable and accurate results, and understand business language or the domain more easily: skipping the frustrations of non-performant AI.
Data governance and infrastructure have traditionally operated in silos, leading to inconsistencies and vulnerabilities. By integrating these two domains, organizations can streamline their data management processes, ensuring that governance controls are applied consistently across all data sources.
This integration also helps in maintaining compliance with ever-evolving data privacy regulations.
📝 Note from Editor: Reference to Solutions on Governance-Infrastructure Integration
The tragedy of Governance failures stems from being “operated in silos”. The infrastructure standard on datadeveloperplatform.org enlists the infrastructure guidelines to ensure end-to-end visibility for governance as if it were loosely coupled and tightly integrated with every entity/level in the data infrastructure: from source to consumption.
Data governance is the backbone of safe AI deployments: the strategic framework and set of practices used to manage data quality, availability, integrity, and security. For AI deployments, data governance plays a critical role in mitigating risks related to data privacy, compliance, and ethical use.
Governments worldwide are increasingly enacting regulations to protect consumer privacy, such as GDPR in Europe and CCPA in California. These regulations require businesses to manage data with care, ensuring that it is collected, stored, and used in compliance with legal standards.
Failure to comply can result in hefty fines and damage to brand reputation. Robust data governance ensures that AI models are trained on data that meets these regulatory requirements, reducing the risk of legal and financial repercussions.
AI systems are prone to biases, which can be exacerbated by poor data governance. For example, if the training data reflects historical biases, the AI system may perpetuate or even amplify these biases in its outputs.
Data governance frameworks that include ethical guidelines and bias detection mechanisms are essential for ensuring that AI deployments are fair and equitable.
With the increasing complexity of data sovereignty laws, where data must reside within certain geographic boundaries, organizations must ensure that their AI systems adhere to these requirements.
Effective data governance includes mechanisms for managing data location, ensuring that AI models only access data that is legally permissible to use.
Tip: Position governance controls as close to the data collection point as possible to enforce standards from the start and minimize the risk of non-compliance.
In many organizations, data producers (those who generate or manage data) and data consumers (those who use data for decision-making) operate in isolation, leading to misalignment and inefficiencies. Encouraging closer collaboration between these groups can enhance data quality and ensure that data is used effectively.
Tip: Create cross-functional teams or regular communication channels between data producers and consumers to align on data requirements and expectations.
🔖 Related Reads
Learn more on collaboration levers and single point collaboration management b/w data producers & consumers: Role of Contracts in a Unified Data Infrastructure
AI itself can be a powerful tool in enhancing data governance. By automating tasks such as data quality checks, metadata management, anomaly detection, and access control, AI can help scale governance efforts and reduce the burden on human teams. This not only improves the accuracy and reliability of your data but also allows your data teams to focus on higher-value activities.
For example, AI models can be trained to identify and flag potential data quality issues before they impact downstream processes. Or recommend standard access SLOs usually implemented at the specific domain/use case level. Similarly, ML algorithms can be used to automatically classify and tag data based on predefined governance rules, ensuring that sensitive data is appropriately handled.
However, it is important to note that AI/ML models used in data governance must themselves be governed by strict quality controls. This includes regular auditing, validation, and updating of models to ensure that they continue to perform accurately and fairly over time.
Tip: Start with small, pilot projects that use AI to automate specific governance tasks and gradually expand as you see success.
🔖 Related Reads
Learn more on how AI augments Governance (quality, security, semantics/experience) capabilities.
AI deployment should not happen in a vacuum. It must be closely aligned with broader business objectives to deliver real value. This means understanding the specific use cases where AI can make the most impact and ensuring that your data infrastructure and governance practices are tailored to support these goals. Avoiding the pitfalls of risky or ineffective AI deployment requires a proactive approach centred on data quality, governance, and collaboration.
Tip: Regularly review and adjust your AI strategy to ensure it remains aligned with your evolving business needs and external factors such as regulatory changes.
AI has the potential to revolutionize business operations, but only if it is deployed effectively and responsibly. By establishing a strong data foundation, integrating governance with infrastructure, fostering collaboration, leveraging AI for governance, and aligning AI with business goals, organizations can unlock the full potential of AI while minimizing risks and compounding costs.
As AI continues to evolve, these practices will become even more critical in maintaining a competitive edge and driving sustainable growth. In fact, they ensure a much more lean delivery compared to kicking off AI without getting the fundamentals right, blinded by the promise of quick surreal results.
Thanks for reading Modern Data 101! Subscribe for free to receive new posts and support our work.
Find me on LinkedIn | Explore my org, MetaRouter
From The MD101 Team
Here’s your own copy of the Actionable Data Product Playbook. With 400+ downloads already and quality feedback, we are thrilled with the response to this 6-week guide we’ve built with industry experts and practitioners. Stay tuned on moderndata101.com for more actionable resources from us!