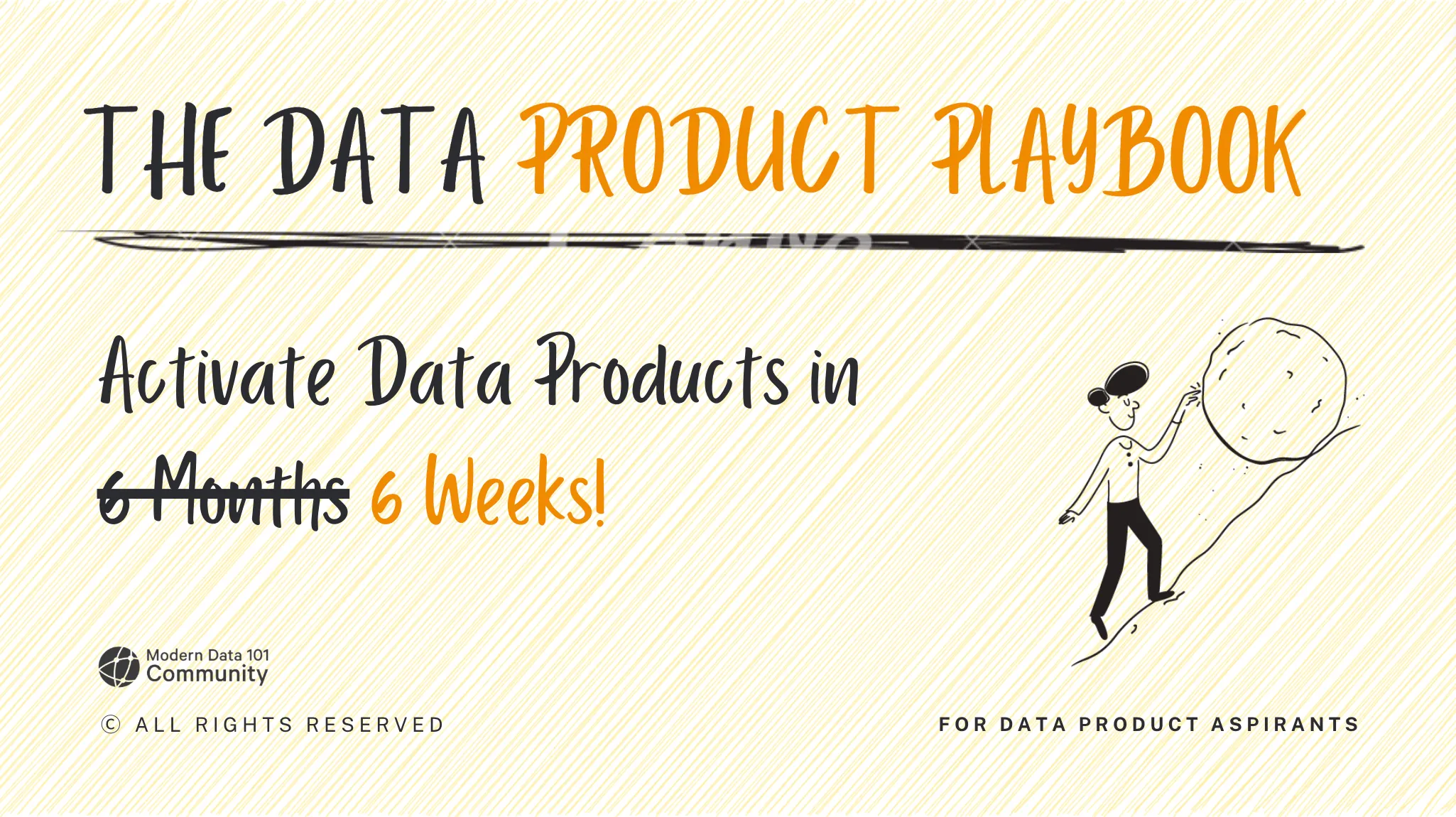
Here's your Playbook
Download now
Oops! Something went wrong while submitting the form.
Learn More
TABLE OF CONTENT
Get weekly insights on modern data delivered to your inbox, straight from our hand-picked curations!
TOC
- Introduction
- Data Product Owner vs. Data Owner: Roles and Differences
- The Risk-Based Franework for Democratized Data Governance
- Conclusion
If we were to compare the situation that typically exists in any integrated company, we could reason that the data is managed centrally by people, classically, and unfortunately, the Ts who do not hold the actual functional ownership. Therefore, unfortunately, we have a paradigm that we could define as data tyranny since a few manage the information, even without having real accountability.
Data Tyranny: A highly centralized governance model that is rigid, formal, and slow to scale. It resembles a wartime economy or authoritarian regime characterized by strict rationing of data resources and limited autonomy; uncertified data are not eliminated but are circulating in a parallel Black Market.
The starting point of our solution must aim for an objective that balances the two solutions. We call it data democratization.
Data Democracy: Governance is tiered, context-aware, transparent, and efficient. It mirrors a mixed market economy, balancing regulatory oversight with innovation and responsiveness, facilitating trusted yet agile data utilization.
Therefore, within operational data democratization, varying levels of quality, control, and verification for products and systems are achievable in a federation with a distributed ownership perspective. No one entity rules all.
To obtain these results, two major achievements have to be performed:
📝 Related Reads
How to Create a Governance Strategy That Fits Decentralisation Like a Glove
The traditional Data Owner ensures data quality, regulatory compliance, and integrity within a specific domain. In contrast, the Data Product Owner has a broader mandate. This role entails end-to-end responsibility for a particular 'data product,' which can be a specific dataset, a data service, or a data application, from identifying requirements through publication and ongoing monitoring.
As an authentic product manager for data, the Data Product Owner addresses technical aspects, usability, transparency, and alignment with end-user operational needs. Their primary goal is making data accessible, reliable, and business-relevant, fostering cross-domain collaboration, and maintaining continuous feedback loops with data consumers.
📝 Related Reads
The Skill-Set to Master Your Data PM Role | A Practicing Data PM's Guide
Transitioning from centralized to democratized governance involves adopting a proportional risk-based governance model. Not all data require the same scrutiny and validation—the level of oversight depends on data sensitivity, the target audience, and the intended use.
For example, datasets intended for exploratory analysis may tolerate higher risk levels than those used for regulatory reporting. This model demands accurate data classification through clear, comprehensive metadata detailing origin, purpose, accuracy, and usage constraints. This transparency allows users to autonomously determine data suitability for specific purposes autonomously, fostering shared and informed accountability.
We could consider the following drivers:
This risk-based certification model allows governance to scale while maintaining control by aligning approval efforts to real exposure rather than applying uniform scrutiny. To operationalize this process, organizations should define clear approval SLAs per risk class, ensuring predictability and transparency:
With these conservative thresholds, the model establishes a straightforward guideline: data products entirely of low-risk drivers, with no more than two medium-risk elements, are eligible for automatic self-approval. The risk is deemed low enough to be managed through standard metadata and platform controls without manual intervention.
A limited review is activated when there are more than two medium-risk drivers, indicating moderate complexity that necessitates a quick governance check—typically focusing on retention, access (review access policies), or source traceability (leverage data product lineage).
However, even a single high-risk driver, such as raw personal data, extra-jurisdictional transfers, or indefinite retention, immediately directs the product into a full governance review. This guarantees robust oversight for sensitive cases without delaying routine, low-impact releases.
A traditional swim lane is something like this:
With a risk approach more towards the left (closer to source), we are positively impacting the project timeline, and also adding time to receive remediation and actions.
📝 Related Reads
Data Governance 3.0: Harnessing the Partnership Between Governance and AI Innovation
Data democratization represents a significant evolution from traditional, centralized data governance frameworks—what we've termed "data tyranny"—towards an agile, transparent, and responsive data environment. This democratized approach increases organizational efficiency and innovation and empowers a broader base of users to leverage data effectively in daily operational decision-making.
Introducing a Data Product Owner clarifies ownership, accountability, and practical data usability, distinguishing clearly between the strategic accountability of Data Owners and the product-centric role of Data Product Owners. By adopting a risk-based governance framework, organizations can ensure proportional and context-aware oversight, dramatically reducing bureaucratic bottlenecks without sacrificing necessary safeguards.
Moreover, democratizing data governance does not equate to deregulation but instead provides a structured yet flexible environment where governance mechanisms are tailored to actual business risks, use cases, and consumer profiles. This balance is fundamental to a vibrant, innovative, and competitive data ecosystem capable of proactively addressing the challenges of an increasingly data-driven market landscape.
Thanks for reading Modern Data 101! Subscribe for free to receive new posts and support our work.
📝 Keep Diving Into Modern Governance Insights
Solve Governance Debt with Data Products
How to Turn Your Data Team Into Governance Heroes🦸🏻| Tiankai Feng
Governance for AI Agents with Data Developer Platforms
Role of Interoperability in End-to-End Data Governance | Issue #43
Challenges of Multiple Data Products, Duplication Management, and Governance | Issue #41
If you have any queries about the piece, feel free to connect with the author(s). Or feel free to connect with the MD101 team directly at community@moderndata101.com 🧡
Find me on LinkedIn 🤝🏻
Some cool new insights dropping soon!