New Release
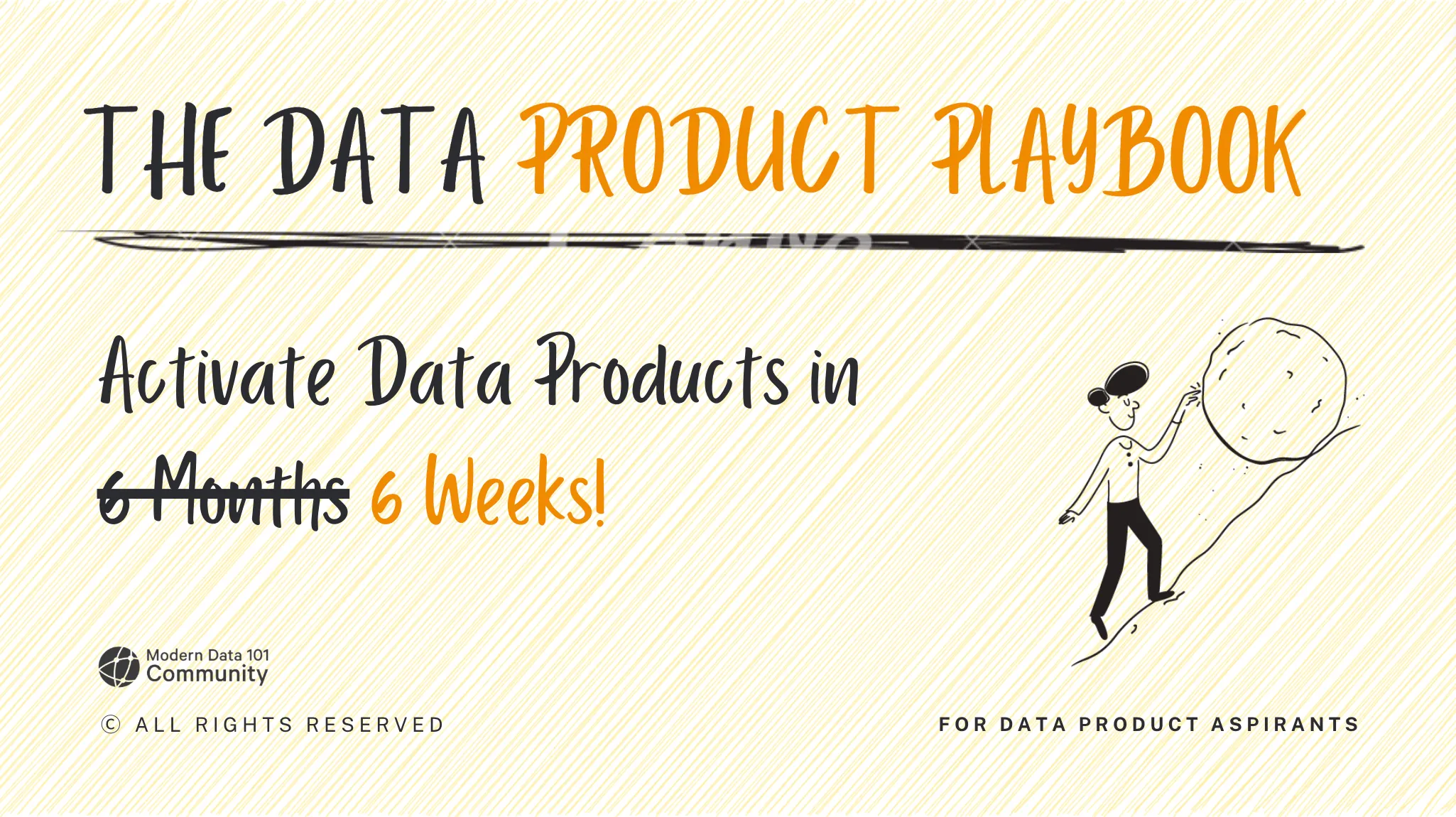
Learn More
Your submission has been received!
Thank you for submitting!
Thank you for submitting!
Download your PDF
Oops! Something went wrong while submitting the form.
Table of Contents
Get weekly insights on modern data delivered to your inbox, straight from our hand-picked curations!
the following is a revised edition.
A couple of years ago, A16Z released a report highlighting the explosive growth of the data infrastructure market, indicating propelled investments in the same space. The report also discussed unified data infrastructure that combines various tools and best practices principles to manage data and the emergence of capabilities like data intelligence and machine learning.
Until late 2020, giants like Google, Microsoft, Snowflake, and Databricks have been serving their clients with a very limited view of the data, and dark data remained a consistent problem with practically no solution at bay.
The report acted as a blueprint for the creation of a cloud-based modern data infrastructure that caters to different organizations and solves targeted use cases giving a complete view of the data to the users.
Years passed, but it seemed the industry seemed to lack concrete efforts in the space. There’s been a lot of dialogue around it, but real action was missing until recent developments surfaced. Data engineering space experienced an overhaul with mergers, acquisitions, collaboration, the emergence of new tools and capabilities, and extensive funding around capability building.
Giants in the space acquired startups and developed capabilities or offered extensions to existing ones. The market took an amusing turn when players like Snowflake and Databricks donned warrior hats and came to loggerheads with each other claiming to have created what seemed to be on the roadmap of a unified architecture.
The industry took a steep turn when Databricks announced the Databricks Lakehouse. The platform construct is said to address the data concerns and mature into an “ideal data architecture for the new era where data, analytics, and AI are converging.” Around the same time, Snowflake started dialogues about The Snowflake Platform. The company claimed that the offering is a “flexible architecture and no data silos platform.”
Meanwhile, companies like Google and Microsoft recently made public announcements about creating a product that is claimed to be more of a fabric and mesh architectural design implementation to solve giant big data problems.
Google defines Dataplex as “a data fabric that unifies distributed data and automates data management and governance for that data”. It is referred to as “the central place to discover, curate, unify data sans movement, organize, govern, and monitor.” Microsoft upped its game by positioning its product, Fabric, as the AI-powered platform. Microsoft claims that the platform “offers a comprehensive suite of services, including data lake, data engineering, and data integration, all in one place.”
All of these are principally on similar lines, but are these solutions truly unified to solve data concerns, or are they merely packaged integrations glued together?
Is anyone else in the market working in this direction? Plenty of emerging Silicon Valley startups have been talking about creating an “ideal unified data infrastructure.” Some have served their clients with integrated capabilities that truly solve enterprise big data problems, while there have been leaders in the space for close to a decade, like ThoughtWorks. They have been talking about creating data mesh and data products for quite some time now and have served numerous clients with this ideology. Both of these designs have certain infrastructure requirements that must be ticked off.
A lot of conversations have taken place about Data Mesh and Data Products on a number of forums. Data Products are the fundamental building blocks of not just a data mesh design framework but can equally enable other such data design frameworks. The Data Products essentially need a unified data infrastructure which is much more than a packaged integration. A packaged integration misses true unification and doesn't carry its inherent benefits, such as unified governance, universal metadata and metrics, right-to-left data engineering, and so on.
This has been achieved with the advent of the Data Developer Platform (DDP) infrastructure specification, which is a community-driven open specification for establishing a unified infrastructure. Platform developers can contribute, enhance, and/or adopt the specification to build their infrastructure from the ground up. Having said that, Platform developers can also embrace existing infrastructures developed with the DDP specification at its core.
The Data Developer Platform infra spec abstracts complex and distributed subsystems and offers a consistent outcome-first experience to non-expert end users. A Data Developer Platform (DDP) can be thought of as an internal developer platform (IDP) for data engineers and data scientists. Just as an IDP provides a set of tools and services to help developers build and deploy applications more easily, a DDP provides a set of tools and services to help data professionals manage and analyze data more effectively.
A DDP typically includes tools for data integration, processing, storage, and analysis, as well as governance and monitoring features to ensure that data is managed, compliant and secure. The platform may also provide a set of APIs and SDKs to enable developers to build custom applications and services on top of the platform. The primary value add of a DDP lies in its unification ability- instead of having to manage multiple integrations and overlapping features of those integrations, a data engineer can breathe easily with a clean and single point of management.
In analogy to IDP, a DDP is designed to provide data professionals with a set of building blocks that they can use to build data products, services, and data applications more quickly and efficiently. By providing a unified and standardized platform for managing data, a data developer platform can help organizations make better use of their data assets and drive business value.
A DDP for data can be considered to be like an operating system for data. Just like operating systems such as Windows, iOS, Linux, and Android serve users by abstracting the complexity and projecting applications, an operating system for data abstracts and delegates maintenance to the infrastructure so developers can focus on data and data applications that rake in the real value. The objective is to unify and simplify data ops and consumption for data users, ideally data engineers and data analysts. Users no longer need to spend countless hours on plumbing and instead focus on what really matters - the data.
More on unified data infrastructure and DDP:
Evolution of the Data Stack: The story of how we interpret ever-growing data
The Essence of Having Your Own Data Developer Platform
We’ve always had a lot of inspiration from the community and often source resonating ideas from the larger group. So it was high time to create a dedicated space for all the voices that have been shifting the needle and can help us go a step further in our data journey.
Bipin Dayal wrote an exceptional article on LinkedIn where he talks about Transforming Supply Chain Management: The Evolution of Data Architectures. He mentions Data Integration as one of the most prominent issues and how lack of data integration impacts the identification of trends and opportunities:
The modern supply chain generates data from multiple sources, including suppliers, manufacturers, distributors, retailers, and customers. Integrating data from diverse systems and formats into a unified platform can be challenging. Lack of seamless data integration hampers the ability to analyze and interpret data holistically, limiting the organization's ability to identify critical trends and opportunities.
Diogo Silva Santos talks about The Past, Present, and Future of Data Architecture and the generations of data architecture.
The biggest changes from second-generation to third-generation data architecture were the switch to the cloud, the real-time data availability, and the convergence between the data warehouse and the data lake…
Converge the warehouse and lake into one technology, either extending the data warehouse to include embedded ML training or alternatively building data warehouse integrity, transactionality, and querying systems into data lake solutions. Databricks Lakehouse is an example of a traditional lake storage solution with warehouse-like transactions and query support.
At DataOps Day, you’ll take a deep dive into the world of data and DataOps. Join this virtual meeting to hear from data and business leaders who have adopted DataOps to achieve their goals for quality, security and speed.
Speakers Include - Tomer Shiran(Founder - Dremio), Christopher Bergh(CEO - DataKitchen), Venkat Ramakrishnan(VP of Products and Engineering - Pure Storage), Trista Pan(Co-Founder & CTO - SphereEx), Alan Shimel(CEO, Founder - Techstrong Group), and many more such renowned names within the modern data space. Get the full agenda here.
Event Date: August 16, 2023
Mode: Virtual
Register
DataEngBytes Conference is your gateway to an immersive journey beyond the buzzwords, where you’ll dive deep into data engineering's real-world solutions and best practices.
Speakers Include - Ryan Boyd(Co-founder - MotherDuck), Joe Ries(CEO - Ternary Data), Mikiko Bazeley(Head of MLOps - Featureform), Chad Sanderson(Chief Operator - Data Quality Camp), Suneeta Mall(Head of AI Engineering - Harrison AI), Vinny Vijeyakumar(Senior Solutions Architect-Databricks), Abhinav Goyal(Founder - Ordinatim), and many more experienced folks from the modern data space. Check the details on numerous sessions here
Event Date: Perth(22 Aug.), Sydney(25 Aug.), Brisbane(29 Aug.), Melbourne(31 Aug.)
Mode: Offline
Register: Perth, Sydney, Brisbane, Melbourne
As usual, here’s a light breather for you for sticking till the end!
Follow for more on LinkedIn and Twitter to get the latest updates on what's buzzing in the modern data space.
Feel free to reach out to us on this email or reply with your feedback/queries regarding modern data landscapes. Don’t hesitate to share your much-valued input!
ModernData101 has garnered a select group of Data Leaders and Practitioners among its readership. We’d love to welcome more experts in the field to share their stories here and connect with more folks building for the better. If you have a story to tell, feel free to email the Editor!