New Release
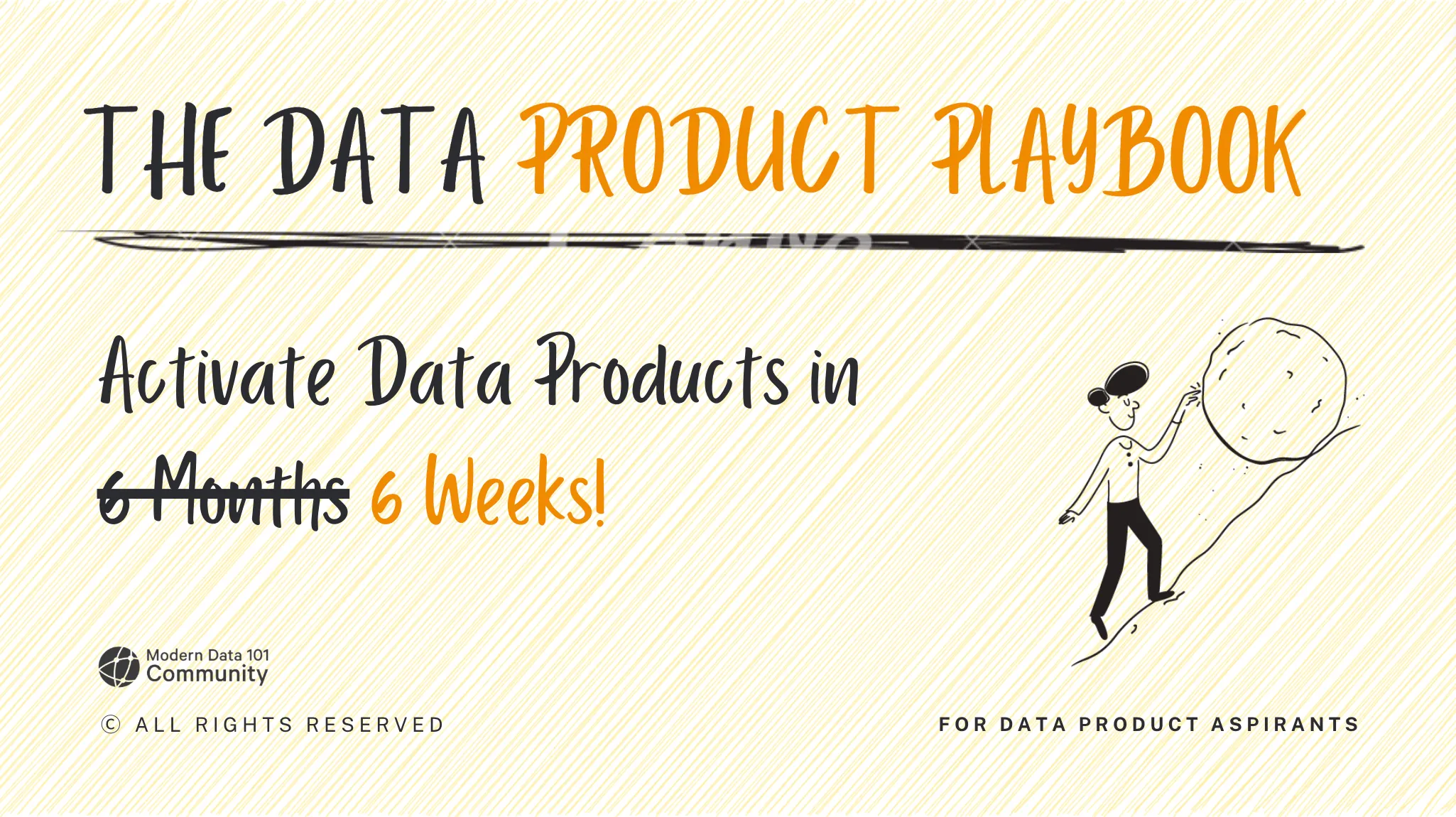
Learn More
Your submission has been received!
Thank you for submitting!
Thank you for submitting!
Download your PDF
Oops! Something went wrong while submitting the form.
Table of Contents
Get weekly insights on modern data delivered to your inbox, straight from our hand-picked curations!
the following is a revised edition.
As we inch closer to bid adieu to a fantastic 2023, it is time to reflect on the dynamic landscape of Modern Data Stacks. The year has been full of groundbreaking innovations and relentless pursuit of data biggies to master the ever-expanding data and data science universe.
Before this year slips like sand, let’s explore the data industry’s corridors and learn about some data trends, breakthroughs, and advancing narratives that defined this year.
The year has served as the centre stage for data zealot companies surfacing major product capabilities. Be it the bold claim of Databricks to be recognised as a Data Intelligence Platform, the numerous collabs the industry witnessed attempting to become a comprehensive data platform or be it the massive adoption of Gen AI (Generative Artificial Intelligence) tools, 2023 will be remembered for good.
Join this attempt to capsulate the evolving industry and hold tight to your seat as this recap takes you for a dive into the deep waters of Modern Data Stacks and everything in and around it.
Let’s jump back into the year-long montage! 🎞️
Initially celebrated for its revolutionary shift to the cloud, the Modern Data Stack inadvertently gave rise to data swamps—chaotic, unmanageable clusters hindering operationalisation. Though MDS was innovative, the industry's struggle with integration overheads and data silos remained unresolved.
In response to MDS complexities, the latest state or the currently “modern” state of the modern data stack emerged as data-first platforms, or what we like to call data-first stacks (DFS). It simply meant data stacks that allowed data developers to focus more on data solutions instead of building and managing data infrastructures. Several Data Developer Platforms, the likes of Keboola, Witboost, and DataOS, came into prominence with an unwavering focus on serving data infrastructure-as-a-service in the form of end-to-end data platforms so data developers can focus on solutions that directly impact business drivers.
The incremental progress of the industry towards Data Developer Platforms was also presented at the Snowflake Summit this year, where the platform was positioned as the single source of truth.
We believe the maturity of organizations in terms of their data platform utilization is evolving rapidly. Our research indicates a dynamic environment where data platforms are progressively diversifying their capabilities.
Data Developer Platforms were also presented at the Data Engineering Study where 22 Data Engineers from 5 companies looked back on the trajectory of 2023 and distilled it down to Data Developer Platforms and Platform Engineering as the natural next step for the data industry.
To learn more about Data Developer Platforms (DDP), check out the independently maintained documentation on datadeveloperplatform.org. It captures the:
and more…
MD101 has also shared an overview of the same:
The Essence of Having Your Own Data Developer Platform
When looking at MDS today, the industry faces challenges in managing complexity and addressing chaos amid innovations. The shift from excitement to practicality reflects a dynamic balance between aspirations and the realities of data landscapes, aiming for a more organised and efficient future for the data industry.
2023 marked the end of the Cold War between Databricks and Snowflake and the beginning of visible confrontations. Yes, the industry witnessed their rivalry going viral. Be it hosting their conferences on the same dates, leaving several enthusiasts confused and surprised or the swift changes in the narratives of these competitors.
Databricks made a bold move claiming to be recognised as a Data Intelligence Platform, indicating the high degree of AI integration on its data platform. On the other hand, Snowflake is tightly knitting AI/ML on the data cloud platform, enabling the building and deploying of Large Language Models (LLMs) and Machine Learning Models (ML Models). However, these aren’t the only ones doing so.
There was notable dialogue on the same from community experts such as Ethan Aaron, Oliver Molander, Neelesh Sailan, and more; all of which saw significant engagement from the data community. Most interestingly, even the founders of these giants jumped into the dialogue as a result of the recurring comparisons.
The year witnessed a number of key players like Alation, Oracle, and Collibra moving forward with adopting the Data Intelligence Platform narrative. As the calendar unfolded, the race to enable their customers to chop the fluff and reach the most value-driving data with the speed of light, if not more, intensified.
As the year advanced, differential definitions of Data Products emerged from every corner of the data industry’s corridors. Data Products became the most relevant character of the play, grabbing the attention of industry experts. However, the concept of data-as-a-product is relatively old; the recent advancements in tech and infra have given it a new life.
Several key players changed their narratives to align with the Data Product approach. Many big-league organisations embraced the shift in narrative and chose to adopt the Data Products strategy to stay ahead of the curve.
Among several distinguished voices in the industry, some very insightful perspectives have emerged on the data product philosophy. Renowned for their grasp of the field, Sanjeev Mohan, Xavier Gumara Rigol, Jon Cooke, Andrea Gioia, and our very own Animesh Kumar lent their expertise, solidifying their faith in the construct and further collectively shaping the ongoing conversation and fostering a deeper understanding of the subject. Feel free to check out some of their materials below:
1. What is a Data Product by Sanjeev Mohan
2. A series of resources from Animesh Kumar & the MD101 community on building End-to-End Data Products
3. A series of POVs on interoperability, semantics, and more from Andrea Gioia
4. A practitioner’s POV on treating Data as a Product at his org by Xavier Rigol
🎯 End-to-End Guide to Building Data Products
Advancing the narrative, Monte Carlo developed a Data Product Dashboard in its Data observability suite, giving data teams a window into the health and reliability of the tables, among other capabilities.
Meanwhile, Ellie led the way in Data Product design and collaboration tools, offering a platform that empowers global businesses to deeply understand and validate their data product needs before committing to costly data pipelines.
As a journalistic piece, it’s imperative to highlight the capabilities of DataOS on this front as well without any intended bias. DataOS has consistently stuck to the data-as-a-product pillar since its inception and currently offers an end-to-end data platform to design, develop, deploy, and evolve data products with your existing data ecosystem.
Several Data Product facilitators have popped up over the recent hype cycle, but in essence, the ones that will stick are not repackaged solutions but ones that are truly treating data as a product.
Advancing the Data Product narrative, key players in the industry have entered into strategic partnerships and even acquired some emerging startups to leverage Artificial Intelligence and Machine Learning (AI/ML) capabilities.
A number of companies seem interested to embed AI/ML capabilities into their product, making them more consumable for business users. Some companies also aspire to embed ChatGPT-like capabilities so that the user can interact with their organisational data from UI without having a deep understanding of coding.
In the last year, data contracts have become a significant trend in the data industry, providing a fresh method to guarantee large-scale data quality and governance in production. By principle, data contracts refer to agreed-upon specifications and expectations on how data should be produced, consumed, and processed, facilitating seamless integration and interoperability among various tools and platforms within the data ecosystem.
Any data ecosystem typically includes components like data warehouses, data lakes, ETL (Extract, Transform, Load) tools, and BI (Business Intelligence) platforms; adhering to data contracts becomes crucial for ensuring consistency and reliability across the entire data pipeline and avoiding siloed solutions. Contracts, again, being a relatively old construct, have gained new attention due to recent needs and innovations. When integrated with the powerful capabilities of GenAI, AI and ML, Data Contracts became more important than ever.
For a quick view of the major breakthroughs this year in the data contract space, here’s a community roundup:
The Data Contract Pivot in Data Engineering
Alongside the highlights in the above piece, Andrew Jones also maintains a great archive of resources around contracts and this 101 on contracts by Jean-Georges Perrin is also quite illuminating.
Towards the end of 2022, ChatGPT made its impactful entrance, transforming the AI landscape with unprecedented GenAI capabilities. Yet, 2023 stands out as the epoch when GenAI tools ascended to prominence. This pivotal year witnessed an unparalleled surge in the utilisation and recognition of these cutting-edge technologies, solidifying their role in shaping the future of artificial intelligence.
Artificial Intelligence at large became the talk of the town, with industry observers, technology enthusiasts, and the general public starting to have dinner-table conversations about the capabilities of the field. The numerous mergers, acquisitions and collaborations with enterprises attempting to up their game also shared the limelight.
Generative AI is a multi-billion dollar opportunity for many! But, how to leverage this technology?
Steve Nouri shares an interesting take on the above which turned many heads. On the other hand, Vin Vashishtha decided to point out a darker angle and what we really need to focus on when it comes to GenAI use cases.
While Google introduced Bard, Databricks surfaced its willingness to delve deeper into AI through strategic collaboration with Nvidia. Hightouch recently acquired Headsup to bring its AI/ML capabilities to its conversion engine designed for product-led growth. As a result, Hightouch will be able to create comprehensive Customer 360 profiles directly within data warehouses.
Meanwhile, Meta made its Llama 2 foundation chat model available to Databricks, allowing centralised governance in the Unity Catalog as well. Meta intends to extend the offering to Snowflake, Microsoft, and Atlassian in the near future.
Leaning on the potential of AI, Snowflake recently announced its fully managed service, Cortex. Cortex is an AI tool designed to help business and developer profiles work seamlessly, making interactions easier and faster with the help of this tool. Also, Cortex will help build GenAI applications on top of the data stored in the Snowflake.
Alation recently launched a GenAI model, ALLIE AI. The LLM is supposed to help organise data while it is being ingested. It also allows streamlining and simplifies finding the right data within the Atlation data catalog. With ALLIE, Alation upped its game in GenAI. The company is training the LLM on the customers' data, thus offering help in the data governance tasks.
As the industry continues to evolve, substantial funding has flowed into the sector, shaping the future of businesses. During the past year, startups and established firms secured significant investments, underscoring the industry's potential and accelerating the transformation of data ecosystems.
Tabular secured $26 million in a recent funding round. The infusion of capital will fuel Tabular's ambitious plans to expand its product suite, with the overarching goal of liberating enterprises from the shackles of 'data lock-in.' This means providing organisations the flexibility to adapt their data architecture in response to evolving needs and emerging technologies.
Databricks secured investments from Nvidia and Capital One, among others, as they poured in more than $500 million in fresh capital. This became more important as Databricks and Nvidia are inclined to deepen their AI ventures.
Seattle-based Gable.ai emerged from stealth with a staggering $7 million seed funding. The company aims to bridge the gap between software engineers and ML developers by providing high-quality, reliable, complete and accurate data flowing into AI applications.
Hugging Face, the AI startup, recently raised $235 million in a Series D round, receiving backing from tech giants like Google and Amazon. The funds will support expansion into research, enterprise, and startups. Concurrently, Ikigai Labs secured $25 million, fueling its mission to innovate AI solutions for tabular data and transform enterprise AI usage.
In conclusion, the year 2023 has been a remarkable chapter in the evolution of the broader data engineering landscape. While challenges persist, the ongoing pursuit of data excellence remains unwavering.
As we navigate the complexities of the data universe, one thing is clear – the transformative forces at play are reshaping not only the way we handle data but also the very foundations of decision-making in the digital age.
The year 2023 serves as a pivotal milestone in this ongoing narrative, and as we step into the future, the lessons learned, and the innovations witnessed will undoubtedly continue to guide the trajectory of the data engineering domain.
What was 2023’s highlight for you?
Feel free to share your thoughts, posts, or resources you found intriguing!