New Release
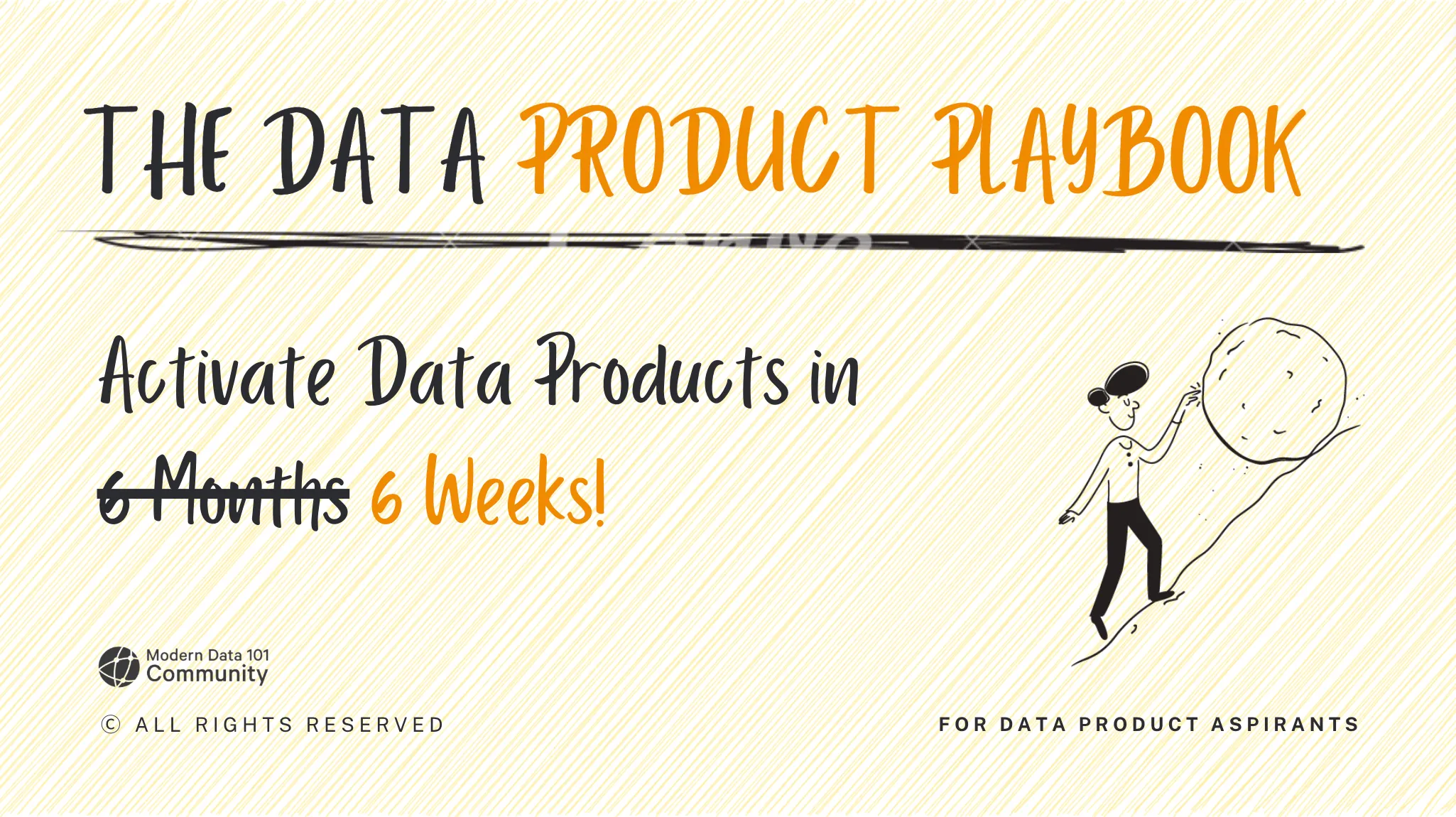
Learn More
Your submission has been received!
Thank you for submitting!
Thank you for submitting!
Download your PDF
Oops! Something went wrong while submitting the form.
Table of Contents
Get weekly insights on modern data delivered to your inbox, straight from our hand-picked curations!
the following is a revised edition.
Game theory has undergone several iterations over the years to arrive at a stage where it almost resembles a “theory of everything” when it comes to competing human ecosystems in any field—be it economics, science, or even data.
Game theory, branch of applied mathematics that provides tools for analyzing situations in which parties, called players, make decisions that are interdependent.
This interdependence causes each player to consider the other player’s possible decisions or strategies when formulating a strategy. A solution to a game describes the optimal decisions of the players, who may have similar, opposed, or mixed interests, and the outcomes that may result from these decisions.
~ Britannica
Two interesting peaks in this theory were 1/ centralisation and 2/ giving control to independent entities.
While the centralisation theory failed the test of time, the latter became the proven standard as the most optimised path to solving problems in competitive settings. Here’s an excerpt highlighting this stark contrast between the two schools of thought in game theory presented by John von Neumann and John Nash.
Players would have to form coalitions, make explicit agreements, and submit to some higher, centralised authority to enforce those agreements - Von Neumann’s superlative instincts failed him. Where von Neumann’s focus was the group, Nash zeroed in on the individual and, in doing so, made game theory relevant to modern ecosystems.
Nash created a theory of games in which there was a possibility of m utual gain. His insight was that the game would be solved when every player independently chose his best response to the other player’s best strategies.
~A Beautiful Mind, Sylvia Nasar
Nash Equilibrium is reached when both players choose to cooperate and share the rewards. However, in reality, players are more likely to act in their self-interest and not cooperate, which can lead to suboptimal outcomes. Nash Equilibrium can be used to analyze complex systems where multiple players interact with each other, such as financial markets, the environment, and social networks. It can help policymakers and researchers to design better policies and anticipate outcomes in these systems.
~ Examples of Nash Equilibrium in Real Life, FasterCapital
Imagine you and your friend are playing a game where you both get to choose a toy to play with. You have two toys to choose from: a toy gun or a race car. The trick is that if you both pick the same toy, you both get to play happily, but if you pick different toys, no one gets to play.
Now, the Nash equilibrium is like a special moment when you and your friend figure out which toys to choose so that neither of you wants to change your choice. For example, if both of you pick the toy gun and are happy, no one will want to switch to the race car because that would stop the fun.
So, the Nash equilibrium is when you and your friend make the best choices you can, knowing what the other person is choosing, and no one wants to change their mind. You're both happy with the toys you picked, and the game keeps going without anyone feeling left out!
Let’s zoom in and bookmark for later🔖: Possibility of mutual gain. The game would be solved when every player independently chose their best response to the other player’s best strategies.
As the eccentric nature of mathematics goes, Game theory ended up as an applied theory beyond just numbers and the mathematics domain. It became a theoretical framework to conceive social situations among competing players. Its standard intention is to produce optimal decision-making for independent and competing actors in a socio-cultural setting.
In any data ecosystem, every domain competes for resources, value, and data. While on paper, we want to describe the organisation as a collaborative ecosystem, the practical reality is very different.
Traditionally, all organisations have been limited by centralised systems. All domains, be it marketing, sales, ops, or HR, have to iterate with a central data team for any data-related use case- the volume of which is steadily rising across all industries.
Every domain team believes their requirements to be most urgent and of the highest priority, and the central team, overwhelmed, often fails to satisfy them all.
The centralized system appeared logical—just like in the early iterations of Game Theory when it was believed that players should form coalitions, submit to a higher authority, and allow that authority to enforce agreements (reminds of domains as coalitions and higher authority as central data teams?).
This was Von Neumann's world, where groups worked as a single entity. However, this approach collapsed under its own weight in modern organizations, just as it did in game theory. The sheer complexity of managing interdependent domains made it impossible for the central data team to respond with the agility and precision required.
📝 Related Read
The von Neumann Bottleneck
Nash argued that for optimal solutions, every player should independently choose their best strategy while considering the strategies of others. This gave rise to the concept of Nash equilibrium—a point where no player could improve their outcome by unilaterally changing their strategy as long as everyone else’s strategies remained the same.
In the context of the organization’s data ecosystem, the analogy was clear: instead of waiting for the central data team, each domain should be empowered to make independent data decisions. This decentralization allowed each domain to craft its own data products—specific data sets tailored to its needs, available at its fingertips (reminds of the Data Mesh?).
Complete decentralization wasn’t practical. While each domain now had control over its data, they were still part of a greater whole. They were competing for the same overarching organizational goal, just as players in a game compete but remain part of the same system.
While Neumann’s focus on the group could be more directly compared to completely centralised systems where a central data engineering team ruled them all and suffered the weight of the kingdom, applying the same parallel to domains (decentralisation at a domain level) was equally stressful, if not more.
By “decentralising” at the domain level, each domain is expected to host its own unit of infrastructure, skilled personnel, and design architecture. Essentially, the problems with centralisation are now cut up in parts and replicated across domains. It’s theoretically more manageable, yes, but not scalable, maintainable over long periods of business evolution, or economical. The increase in cost for each domain to host these dedicated resources was a whole different chapter.
On the other hand, a practical data product stack with its feet deep in the painful world of data engineering proposes hybrid decentralisation, which is where hybrid decentralization emerges as the optimal solution.
In an ecosystem with hybrid decentralisation, data autonomy is paired with centralized governance. Every domain can create, control, and optimize its data products, but there are shared resources, platforms, and rules that ensure the entire organization remains aligned. Each domain is independent but not isolated through a common interface, which allows both domains and data products to interoperate.
Here’s where Nash equilibrium comes into play. In this hybrid system, domains operate independently but always consider the strategies of other domains. No domain can gain an advantage by monopolizing data or over-allocating resources because doing so would disrupt the balance. Instead, they optimize for mutual benefit, knowing that the success of one domain contributes to the success of the whole organization.
Instead of each domain/group hosting its own dedicated resources, infrastructure, or skilled personnel, the decentralisation happens at the data and logic-level. This enables the org to share considerable resources and save tremendous costs, all while cutting down the debt and overwhelm of centralised data systems.
Here, the shared resources include:
Examples of artefacts within the boundaries of data products (not shared): Data Product specification, transformation code, port-specific policies or SLOs.
Smart decentralisation = Decentralisation at the data product level (with the domain’s purpose-specific data product specifications).
Smart decentralisation ≠ Independent data stacks and data engineering teams for each domain.
Where von Neumann’s focus was the group, Nash zeroed in on the individual and, in doing so, made game theory relevant to modern ecosystems.
Let’s expand on the idea where data products act as the individuals, and domains act as the groups, drawing parallels with Nash’s game theory to achieve an optimized data ecosystem.
In Nash’s game theory, each player (individual) chooses their best strategy based on the strategies of other players, with the goal of reaching a point of mutual gain where no player can improve their position without affecting the others.
In a hybrid decentralized data ecosystem, the "players" are the data products themselves. Each data product is a self-contained, purpose-built package that delivers insights or value to a specific domain (group).
However, these data products are not isolated. Just as individuals in a game must account for the strategies of others, data products must be designed in a way that complements and interacts with the other data products in the ecosystem.
Domains—such as marketing, sales, or operations—are the larger entities that benefit from the optimal strategy of their individual data products. These domains are similar to coalitions or groups in game theory that have collective interests but must rely on the behaviour of their individual members (data products) to succeed.
Each domain wants its data products to be as effective as possible, but it also understands that its performance is linked to how well other domains are functioning. For example, sales rely on data products created by marketing for lead generation, while marketing needs feedback from sales data products to fine-tune their campaigns.
In a hybrid decentralized data ecosystem, as we saw above, there’s a balance between autonomy (each domain controlling its own data products) and central coordination (overall governance ensuring alignment).
This mirrors the Nash equilibrium—each domain operates independently, but its success depends on how well its data products interact with and support the data products of other domains. Here’s how data products help to achieve the optimized path as suggested by game theory:
Each data product is optimized to solve a specific problem within its domain, allowing for specialization. For instance, a marketing attribution data product helps attribute sales to specific marketing efforts. At the same time, it informs sales forecasting, which is another domain's data product.
The independent specialization ensures that each data product operates efficiently within its scope, much like how Nash’s players optimize their strategies independently.
The Nash equilibrium suggests that mutual gain is achieved when every player independently chooses their best strategy while taking into account the strategies of others.
In the data ecosystem, this means each domain creates data products not only for their internal use but also with the understanding that other domains will use and benefit from them. For example, operations may create a supply chain optimization data product that marketing leverages to adjust campaign timing and resource allocation.
Just as in game theory, where players adjust their strategies based on the actions of others, domains must adjust their data products based on how other domains' data products evolve. This leads to an optimized data ecosystem where each data product evolves over time, improving based on feedback loops from the entire system.
In game theory, while players operate independently, there are rules that govern the overall game to ensure fairness. Similarly, in a hybrid decentralized data ecosystem, each domain is autonomous in managing its data products, but there is a central governance framework that ensures that data products adhere to standards, share common data, and follow best practices. This prevents the chaos of complete decentralization and ensures that the data ecosystem stays optimized across the board.
Nash equilibrium is not static; it can shift as players update their strategies. In the data ecosystem, as business priorities change, data products continuously evolve, with each domain refining its products to align with the organization's shifting goals.
Marketing might focus on brand awareness one quarter and then shift to customer retention, adjusting its data products accordingly. This dynamic adjustment ensures that the entire data ecosystem remains optimized for both short-term and long-term objectives.
The game-theory-inspired model isn’t a new model or data design paradigm. It simply validates the Data Product vision.
Data products function like independent players that adjust their strategies (or design and operations) based on the needs of other data products within the ecosystem. The domains, acting as larger groups, benefit from the mutual optimization of their data products, achieving a Nash equilibrium where:
By leveraging these principles, a hybrid decentralized data ecosystem driven by data products creates an optimized environment where mutual gain is achieved through interdependent, independent data solutions. This results in a system that is agile, efficient, and continuously improving as each domain refines its data products in concert with the others.
Thanks for reading Modern Data 101! Subscribe for free to receive new posts and support our work.
If you have any queries about the piece, feel free to connect with any of the authors (details in Author Connect below). Or feel free to connect with the MD101 team directly at community@moderndata101.com 🧡
Connect with me on LinkedIn 🙌🏻
Connect with me on LinkedIn 🙌🏻
Here’s your own copy of the Actionable Data Product Playbook. With 600+ downloads so far and quality feedback, we are thrilled with the response to this 6-week guide we’ve built with industry experts and practitioners. Stay tuned on moderndata101.com for more actionable resources from us!