New Release
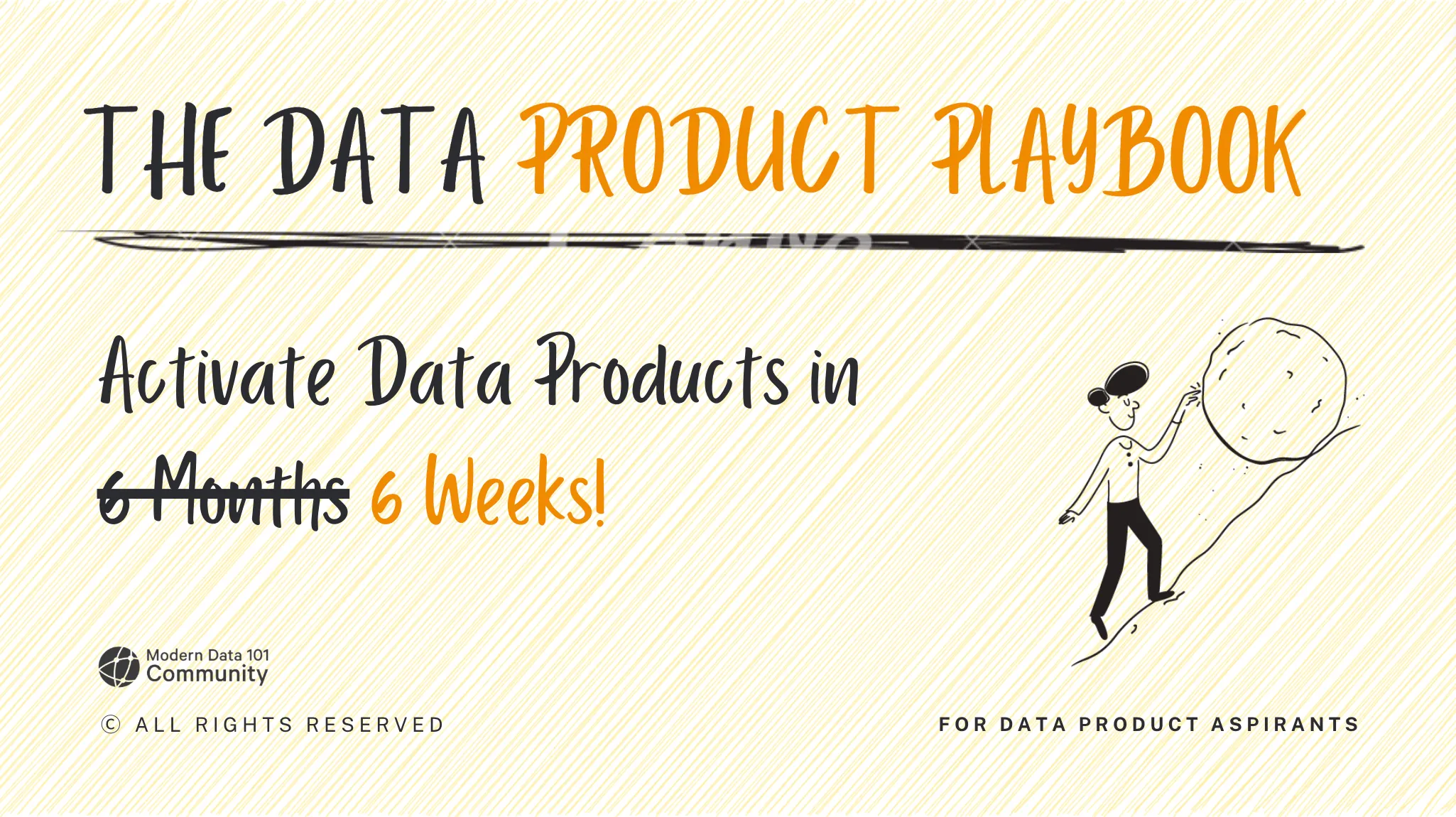
Learn More
Your submission has been received!
Thank you for submitting!
Thank you for submitting!
Download your PDF
Oops! Something went wrong while submitting the form.
Table of Contents
Get weekly insights on modern data delivered to your inbox, straight from our hand-picked curations!
the following is a revised edition.
Immature development and delivery processes force business users to build their own solutions that result in an ever-expanding universe of data silos, intensely fractured data environments, and general data heterogeneity. These examples can be considered as Data Debt, which stems naturally from the way companies do business, especially when they are running their business as a loosely connected portfolio or making “free rider” decisions about Data Management and Governance.
~Petr Travkin, Data Architect
As found by Mckinsey’s extensive report, the majority of the CIOs feel that tech debt has risen since about half a decade ago, which is about the same time when the mass of organizations started leveraging data at scale.
The idea of data was propagated as the new fuel for industries, and several vendors started coming up with a way to manage this fuel without considering that mismanaged data had the potential to make existing infrastructures flammable.
Organizations poured heavy investments into data technologies which were immature solutions that only tried to solve fractions of the huge problem. It was similar to installing a measly pipe to control the direction of a river and continuously fixing the pipe when it ruptured.
Organizations took the natural next step of installing several more of these pipes or point solutions to control and harness the power of the data when instead, they probably needed just one wholistic dam. This chaotic web of tools and their overheads resulted in massive data debts.
Organizations are consistently chasing the unified whole that could govern the entirety of the data ecosystem to make it more reliable. Lower data debt would make it easier for organizations to mine actionable business insights from raw data instead of going around in loops to fix and revive fragile processes and tools.
This article is a snapshot of data debt, the problems it creates for data teams, and an overview of potential solutions that are aligned with DataOps.
To understand data debt, we must first get a hang of technical debt.
Technical debt is the cost of continuous rework when a constructive, challenging, or time-taking approach is replaced with an easier or instant solution to hold the fort. Technical debt rises over time as the reworked jobs and their consequent costs build up.
The concept of data debt is a simple extension of technical debt, where instead of general technologies, the rework and the cost behind the rework are triggered by data-specific technologies. Data debt is often used as a directional measure to understand which problem to prioritize and fix first and it also acts as a directive for investment channels, often deflecting investments instead of harnessing them.
Given the rise of point solutions or multiple pipes that try to control data, the overheads behind each of those solutions have also increased. While the work to install and integrate each of these solutions is already significant, the rework cost triggered by these tools and processes is gradually killing the strategic, innovative, and scalable potential of data teams. Instead of moving forward, teams are stuck in a horizontal plane shuttling between points.
Professionals started dabbling with data only recently whereas software has been under experimentation for decades. As a result, data development is quite scattered and does not necessarily follow the optimized processes that are standard for software development. Sub-optimal processes and approaches are the prime reasons behind the skyrocketing cost of data debt.
Let’s zoom out to an analogy: The tale of the four horsemen. According to the book of Revelation, their appearance brings forth the cataclysm of the apocalypse. The “four horsemen” is an interesting analogy for pre-apocalypse and no doubt, the data world is internally in an apocalyptic stage, given the massive data debt that is being shouldered by weak data pipelines and data teams every day.
The four horsemen of data debt or an apocalyptic combination of processes are currently the common issue in most organizations that are either not data-first or are on the steep and rocky climb of achieving data-first status.
If the above practices or processes are standard in your team or organization, it is evident that the organization is feeding data debt instead of solving it. Data debt results in scores of issues in the data ecosystem, including some of those mentioned in the previous section. but the issues could be categorized into three key pillars:
The most effective way to combat the four horsemen of data debt is to follow the battle cards of Agile development that revolutionized the software industry and most importantly, upgraded the SaaS experience. Agile development is in alignment with the ideologies of DataOps. While DataOps is a data development culture, agile can be described better as a methodology.
The agile manifesto has some key principles such as being change-friendly, enabling early and continuous delivery, and prioritizing users over processes. The next article in the series will outline the top agile principles and illustrate how exactly they could be replicated from the software world to the data ecosystem, gradually eradicating data debt one step at a time.
Since its inception, ModernData101 has garnered a select group of Data Leaders and Practitioners among its readership. We’d love to welcome more experts in the field to share their story here and connect with more folks building for better. If you have a story to tell, feel free to email your title and a brief synopsis to the Editor.